The Importance of an AI Operating Model and Solid Data Foundations (Guest blog by Mesh-AI)
AI is reaching a tipping point. For years it’s been under-realised and under-governed. However, due to its increasingly widespread accessibility, adoption and hype this really needs to change! The availability of such powerful models like the GPT family have caused companies to jump in at the deepest end of AI, without the right foundations in place to both prepare and safeguard for the future. There are two areas that can change this; getting the data foundations right across your business and having an embedded end to end AI operating model.
Let’s unpack this and consider people, process & technology needs for organisations when establishing a future ready AI Operating Model.
AI Operating Model
When most businesses approach AI & ML, they largely focus on model development and deployment. Hence the rise in popularity and adoption of approaches such as MLOps. But, for long term sustained growth and impact, there are five key areas that enterprises must conquer to safely and effectively scale AI in their business. They are:
- AI Culture
- AI Ideation
- AI Development
- AI Trust
- AI Measurement
Getting Started: Establishing an AI Culture
AI Culture is what will drive adoption and impact across the other four areas. Having the right leadership and strategy in place and embedding this at the highest strategic level is key. How you put AI at the core of your message to the wider company, ensures they see how and why it’s a priority for your business and your customers.
In addition, education and literacy are important to bring on board your workforce, finding the balance between the human enablement side and the threat to the future of work as they know it. There is a way to get this right and building a culture around AI will set you, your employees and customers up for long term success.
Generating Big AI Ideas:
AI ideation covers the experimentation areas of AI, however, this should not be a room of scientists off to the side somewhere. This should be integrated with the wider business to source, validate and optimise ideas and output to add lasting business value. This means having a well established AI use case identification process, linked to real business outcomes you are looking to drive. On top of this you need the right technology stack to allow for rapid and robust experimentation with well established and governed data discovery.
They are not often looked at as such, but AI and data are creative enablers by nature, allowing your people to uncover patterns and ideas that open up value drivers for your business. Building an ideation process that allows for AI to be creative alongside your people, whilst having the oversight and direction against business outcomes, will optimise your long term ROI of innovation.
Building Game Changing Models:
AI Development is centred around fully formed and validated ideas. Once you have an AI application with designed outcomes and feasibility checks complete it is taken into development with the ultimate aim to deploy it into production. The well established practice of MLOps plays a large role here but is often overlooked as a pure “technical solution”. I can’t stress enough that this is not the case!
There are two sides to MLOps; organisational management and engineering development. The former is centred around areas of the MLOps process that have integration with wider organisational functions and ensure you are well governed, controlled and auditable in your approach to ML model development. Areas such as; lifecycle management, continuous updates, user feedback, security and KPIs. Framing MLOps in this way ensures you are building the model management solution that results in business focussed outcomes and future proof controls to cope with the rapidly evolving regulatory landscape.
On the other hand, MLOps for engineering development is specific to the machine learning process. More well known areas such as training models, pipelines, registries, evaluation and deployment mechanisms. All important, but without the organisational alignment these robust ML development practices can still fall short of the impact they deserve.
Explainability & Ethics Are Key:
AI Trust is finally getting the attention it deserves based on the recent advancements in Generative AI, highlighting concerns such as ethics, bias and governance of use. However, in many sectors such as financial services and healthcare, this has been a growing focus for quite some time. AI Trust covers both the governance and ethical side of the coin, as well as the explainability of the underlying models. All of which leads to what the industry refers to as “Trustworthy AI”.
Outlining an ethical stance and putting in place the right governance over the entire AI development process is critical. From data sourcing, to training, to evaluation, all the way through to monitoring the deployment of a model. Governance comes in the form of people; roles and responsibilities as well as the technological solutions to enforce this, linking directly to your MLOps framework.
In addition, fairness and bias are evaluation metrics that are key when adhering to well governed AI developments. In my experience this should be applied to both AI you develop internally as well as that you source from third parties.
Explainable AI is more important in some sectors, based on user applications and the types of data involved. It also has varying degrees of difficulty depending on the type of model you are using. For example, the GPT family of models are extremely difficult to dissect and explain how they actually learn.
Striking the right balance of explainability and performance can be difficult but outlining these factors are important to making that right decision. Finally, on the human side, increased trust is achieved by transparency and will ultimately drive adoption and usage across any organisation.
AI Impact is the highest area of uncertainty right now. Prior to November 2022, we would say that huge investments in AI across the board were not yielding the impact they should. Now, with the Generative AI take off, we have an unsubstantiated viewpoint that the impact is unanimous. AI is having a much wider impact, however, most companies are still not putting the right measurement framework in place to quantify the investment and returns.
Data Foundations Hold the Key to the AI Panacea
Putting in place an AI operating model around these 5 areas will ensure your approach to AI is a robust one that optimises business outcomes and mitigates the huge risks that come with it. One key area in particular that underpins the ability to actually deploy such an AI op model, are your data foundations.
Key Areas include:
- Data Accessibility: allowing your AI development teams to find and use the data they need, when it really matters.
- Data Trustworthiness: Ensuring data is well maintained and understood.
- Data Governance: The way in which data could/should be used and how lineage is tracked.
The way in which you enable and enforce the above considerations around data is through a well defined data strategy and modern Data platform approach. Typically this should be componentized and where possible as modular as possible. A distributed, decentralised and domain driven foundation will allow your organisation to map data to business structures and outcomes, in a way that is both accessible and well managed.
A federated data governance approach also allows autonomous data domain teams and centralised data governance functions to collaborate in order to best meet the data needs of the whole organisation. Finally, applying product thinking towards the build of your data foundations allows you to focus on the business goal, treating data as a product that will deliver impact as opposed to a consequential bi-product.
AI… Is not Easy. You Need to Take a Proven Approach to Safe Adoption
In today’s world, technology is not the hard part, the design is. With the scale of compute, the availability of software and the ease of implementation, the challenge is to build data foundations that are well aligned and managed across your business and pointed towards future impact.
However, everyone, it seems, is racing at 1,000 mph without fully understanding the implication of AI on their business. As a result, they will at some point in the near future realise that their ways of working, operating models and technology foundations are not capable of scaling to meet the increased demands that AI is placing on their businesses.
This is why Mesh-AI created Orbital
We have invested in our transformation framework Orbital to support customers in their adoption of burgeoning AI technologies. Orbital is a prescriptive outline that enables organisations to take incremental steps on what is still a very unknown journey.
Firstly, by applying our Orbital methodology we enable organisations to raise awareness and understanding on hot topics such as generative AI. Separating fact from fiction and providing customers with a balanced perspective so that they can make the right decisions for their business.
Secondly, we guide customers to establish a shared consensus and vision for how their organisation can apply techniques such as generative AI to tackle compelling business needs and not just execute side-of-desk science projects.
Thirdly, we align new people, process and technology interventions to ensure AI can be adopted safely and securely, whilst protecting their most precious information assets from data leakage challenges. Designing and establishing new ways of working and governance models to align with rapidly evolving regulatory commitments. Establishing end to end traceability across technology platforms, data sets, systems of record and analytical decision making.
Upon establishing a future vision, we then work with customers to provide them with an incubation process where they can test and learn how AI actually operates in their business. Proving or disproving hypotheses in a way that can support longer term investment needs, founded on sound and justified business cases.
This ensures they don't miss other opportunities to apply other forms of AI or advanced analytics in their business to make a real difference to their customers' experience. This is particularly important given the current hype around generative AI. Sometimes the best solution is not the most sophisticated. Indeed, in taking this approach organisations can weave together their modern data architectures, platforms and discovery tooling with MLOps workbenches to rapidly test new ideas in a self-service way.
We then bring each of these constituent parts together and execute Lighthouse Projects, where bleeding edge techniques such as generative AI can be used to build new products and services, whilst establishing new mechanisms to sell to and service customers with generative AI powered solutions. However, what is critical is to ensure that your organisation has the right service wrap, operating model and governance to ensure that AI does not run away with itself. Equally so, you need to provide your people with the opportunity to learn new skills in a manner that protects them from failure, whilst controlling costs and reputational balance.
As I said, AI is not easy. As such, it’s critical to have the right adoption plan, transformation strategy and use cases that enable your business to thoroughly road test how AI can be applied in your business. All whilst maximising your organisation's crown jewels… and that is your own data!
Sounds compelling…? Then reach out today, with our team of Data & AI experts, who can help you build and implement a rock solid next generation AI strategy using our tried and tested Orbital methodology.
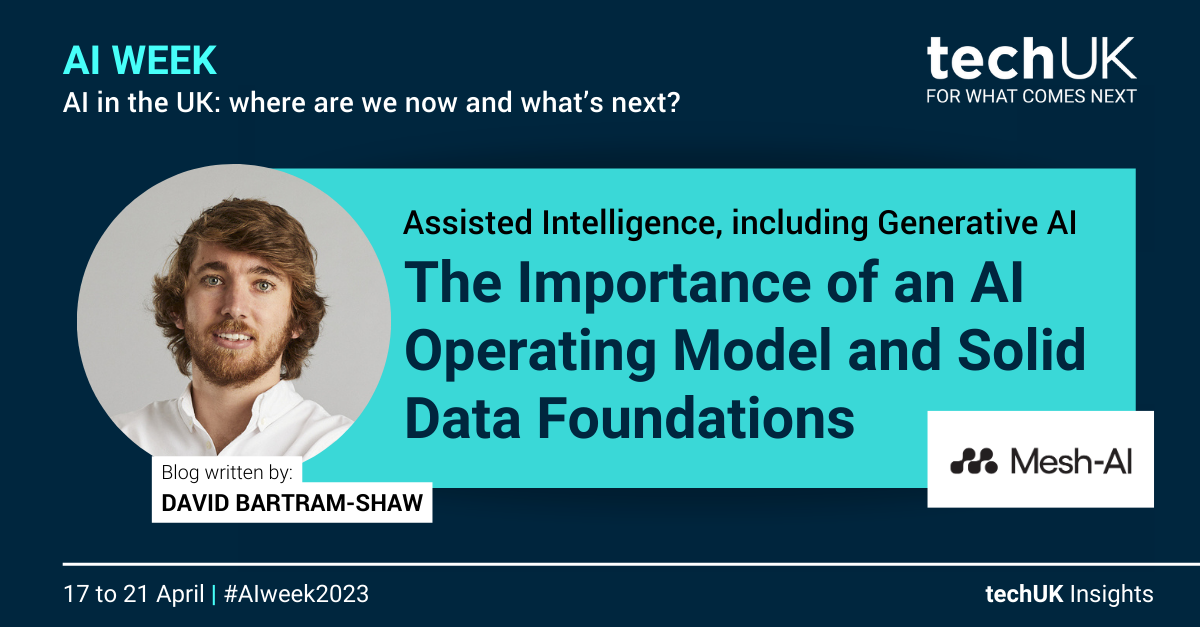
Interested in seeing our latest blogs as soon as they get released? Sign up for our newsletter using the form below, and also follow us on LinkedIn.
Get our tech and innovation insights straight to your inbox
Sign-up to get the latest updates and opportunities from our Technology and Innovation and AI programmes.