KPMG use AI to understand and improve A&E waiting times and support operational decision making (Guest blog by KPMG)
Challenge: Understanding and improving A&E waiting times
The issue being tackled is understanding and improving patient waiting times in hospital A&E. KPMG developed a machine learning algorithm to predict the likelihood of A&E patients waiting more than the 4-hour target and identifying the primary clinical and operational factors influencing the breach.
Solution: AI models and data to support operational decision making
KPMG developed a machine learning algorithm to predict the likelihood of A&E 4-hour target breaches and derive the actionable insights to reduce A&E waiting time. Anonymised patient level records and dynamic features regarding hospital operational conditions were used for model training. Data was stored in Azure blob storage. Extensive feature engineering was performed along with class imbalance techniques for better predictions. The final model was selected after multiple iterations and implemented in Azure ML workspace for predictions.
The AI model aims to provide insights to support operational decision making. They should never be used as an automation or decision-making tool as they are.
Barriers: AI skills and training for healthcare staff
There are couple of barriers to AI adoption in healthcare domain. The successful AI solution requires innovation in integrating software systems to hospitals and involved new processes for doctors, pharmacists, and others to interact which is quite challenging. However, this can be reduced by proper training and user-friendly & easy to understand tools /processes.
Typically, advanced models are neural network based which are considered as black box which are difficult to understand and interpret. This lack of transparency can reduce trust in AI and reduce adoption by health care providers, especially considering that doctors and hospitals will likely be held accountable for decisions that involve AI. Interpretable and Responsible AI might increase trust by eliminating the black box problem, allowing health care workers to understand how AI reaches a certain recommendation.
There are various regulations in healthcare industry like, privacy regulation on patient’s sensitive information and the regulatory approval process for a new medical technology takes time. KPMG has been compliant with these regulations and built these models without compromising patient rights or quality of care.
Impact: Successfully identifying patterns in A&E waiting times
The prediction model for A&E patient waiting time successfully identified 79% of the patients who will breach the 4-hour waiting time target at the point of A&E arrival. Model performance was monitored through area under Receiver Operating Characteristic (ROC) curve, precision and recall.
Model explainability analysis highlighted the important factors impacting these breaches. From patient side, patient age, previous number of admissions, month of arrival, and hour of arrival are the top characteristics contributing to the likelihood of breach. From hospital side, number of breaches in previous hours and the grade and specialism of the attending clinician are the top factors.
For example, the risk of breach is increased by the patient having one or more previous admissions and age. Specifically, infants under 5 years are less likely to breach in contrast to older patients (85+ years) are significantly more likely to breach compared to younger patients. Suggesting a difference in the clinical treatment pathways in A&E for these polemical age ranges.
The model helped the hospital to mitigate the risk of these breaches, improve service delivery and thus patient experience, coupled with reduced financial penalties due to A&E breach.
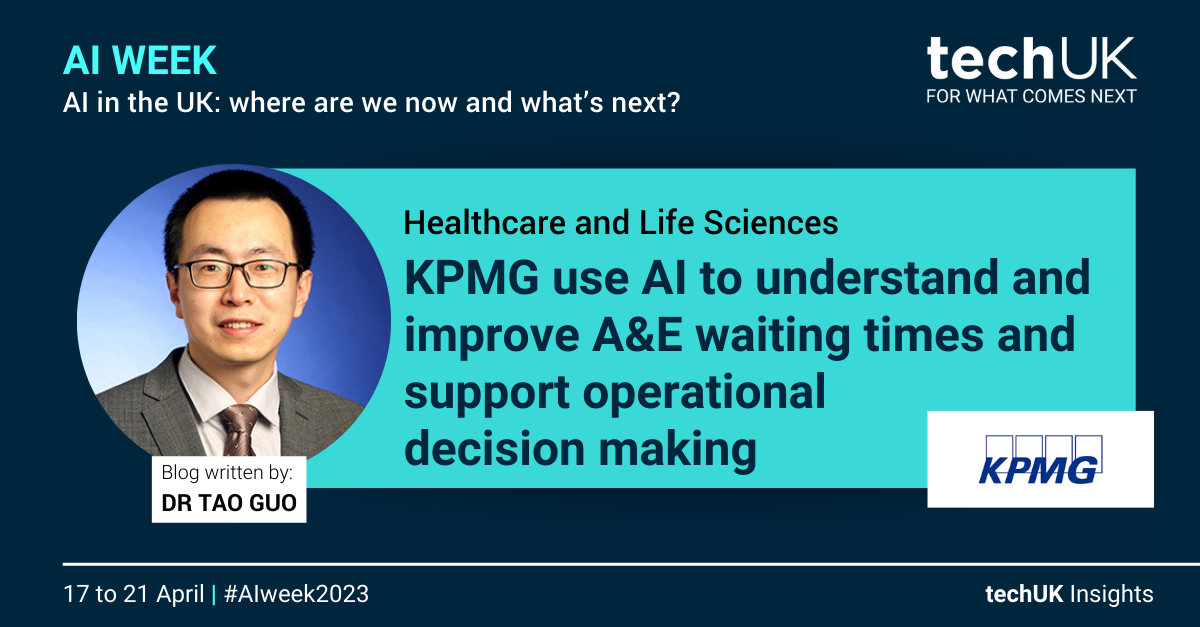
Get our tech and innovation insights straight to your inbox
Sign-up to get the latest updates and opportunities from our Technology and Innovation and AI programmes.