Build AI’s house on rock
Too much emphasis on AI/ML development at the expense of investment in data is like building a fine house on a beach then only later seeing the walls crack and fall as the sand moves beneath it.
The Government’s Data Strategy recognises this when it talks about data foundations as “data that is fit for purpose, recorded in standardised formats on modern, future-proof systems and held in a condition that means it is findable, accessible, interoperable and reusable.”
At DesAcc, we apply our knowledge of medical data to several fields, including medical imaging. The examples below are specific to imaging but highlight challenges that span industries.
AI shows great promise for assisting or even automating the interpretation of X-ray, scan and microscopy images. But the development of AI models safe enough to be used clinically depends on making data findable, accessible, interoperable and reusable.
Firstly, there is a problem in selecting data initially to create cohorts for AI model development. The quality of tagging metadata is often poor. Let's take Body Part as a simple example. This is often incorrectly populated, due to errors in the setup of the acquiring X-ray machines and scanners. Medical imaging uses Digital Imaging and Communications in Medicine (DICOM) as an exchange protocol, and it defines valid values for Body Part. DesAcc’s analysis at one site found Body Part to contain an invalid value in over 50% of exams. Using only valid values to select appropriate exams for AI development would have missed many candidate exams. Good data is already scarce and this scenario makes it scarcer.
Secondly, the same concept is often represented in many different ways – a barrier to interoperability. Magnetic Resonance (MR) scanning is a good example. A key parameter for MR is the timing of radiofrequency pulses. MR scans are usually “T1-weighted” or “T2-weighted” (T1w/T2w) to pick out various tissues and AI developers often want only one kind for model training and testing. Radiologists have learned to easily distinguish these by eye but a computer relies on tags. Each scanner manufacturer and even hospitals use different tags and naming conventions, making it very hard for machines to pick out just T1w or T2w images.
Thirdly, AI models in imaging don’t just learn from the images; they need to be told what they’re looking at. This means providing “labels” along with the images.
Good quality labels often have to be created by several expert radiologists, an expensive and scarce resource. Attempts have been made to auto-create labels from the written diagnostic reports but Dr Luke Oakden-Rayner’s research highlights concerns about re-using labels provided with an extensively used chest X-ray dataset.
Furthermore, the most granular detail about what’s in an image often resides in another system. For cancers and many other diseases, the microscopy performed on tissue removed from the patient is the “gold standard”. That’s contained in the pathologist's report of what was observed under the microscope. Access to the systems that hold it is often an issue, and interoperability is hindered by a lack of interoperable data in a machine-readable format.
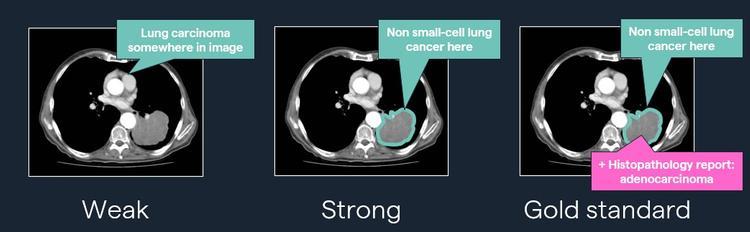
Demonstration of different levels of image labelling
This report by Ernst and Young reiterates the Data strategy’s point about the value of combining and linking datasets from different systems when it comes to developing machine learning models.
All these issues contribute to a shortage of quality data in medicine. Given that AI algorithms are, at their heart, statistical models which make predictions from given inputs, it’s not hard to see that a bigger and more diverse pool of data for training and testing will help reduce the risk of bias and false results.
DesAcc believes that investment to improve our data foundations across all sectors will yield great dividends. Data quality and quantity must be the rock that the UK’s AI strategy is built on, no matter what the industry.
Author:
Graham King, Product Manager, DesAcc EMEA Ltd
You can read all insights from techUK's AI Week here

Katherine Holden
Katherine joined techUK in May 2018 and currently leads the Data Analytics, AI and Digital ID programme.