Intelligent Automation to ease the Data Cleansing burden
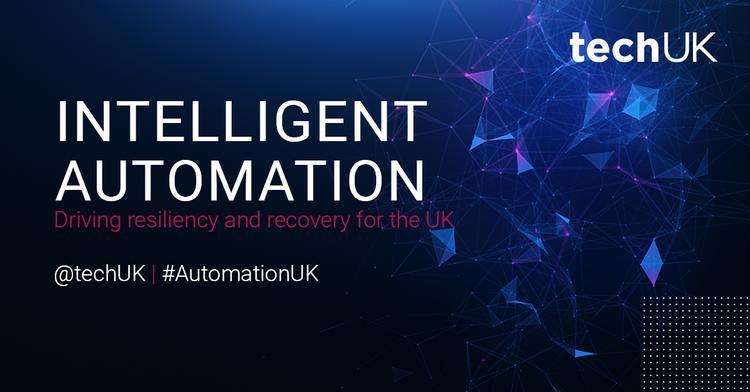
In common with much of the public sector, the technology estate across the Ministry of Defence (MOD) is very broad, with a wide range of legacy systems either MOD-managed or supplier-managed. The various data sets held in these systems are accessed, updated and exploited by multiple teams across MOD and supplier organisations. This inevitably leads to the need for Data Cleansing activities to ensure that the quality of each data set is as high as possible. These activities are usually very labour intensive and time-consuming.
There is significant potential to use Intelligent Automation to assist with this Data Cleansing effort. Using a combination of Machine Learning and Robotic Process Automation (RPA) we can identify poor quality data, make recommendations for the correction and action those corrections on the source system.
Focusing on specific high-impact data quality issues where the criteria for what constitutes good quality data are clear, step one is to create an application for the user to triage each issue identified. This triage (and any record of historic data corrections) builds an evidence set against which a recommendation engine can be trained. Step two is for the application to start suggesting to the user the most appropriate action for each case. The user then either agrees or adjusts the action, providing the necessary feedback loop to improve the accuracy of the recommendations. As confidence builds, the time spent manually triaging each case reduces, allowing the user, as the subject matter expert, to focus on the more complex cases.
While the recommendation engine represents one aspect of Intelligent Automation, and can save time and manual effort, it does not tackle the manual action to correct the data in the source system. Ideally the corrective actions needed would be automated through APIs but given the nature of disparate legacy systems, API access is often not available and not feasible to introduce. In this case RPA can be employed to make the changes, accepting an input from the data cleansing application of the actions required and executing those actions on the source system.
There are several challenges in achieving this, such as identification of suitable subject areas, establishing a training and test data set for the machine learning algorithms, and agreeing access protocols for the RPA agent to the source systems. Additionally, while IA can help to ease the manual burden and improve data quality, it does this in collaboration with subject matter experts not instead of them. However, in specific cases, confidence in the automated recommended actions may be high enough to allow the application to run autonomously.
Will Intelligent Automation solve our all data quality issues across a fragmented and often aged IT estate? Of course not, but it can ease the manual burden, accelerate improvements and allow the experts to focus on the high-value high-knowledge activities.
You can read all insights from techUK's Intelligent Automation Week here

Rory Daniels
Rory joined techUK in June 2023 after three years in the Civil Service on its Fast Stream leadership development programme.

Laura Foster
Laura is techUK’s Associate Director for Technology and Innovation.

Elis Thomas
Elis joined techUK in December 2023 as a Programme Manager for Tech and Innovation, focusing on AI, Semiconductors and Digital ID.